
[ad_1]
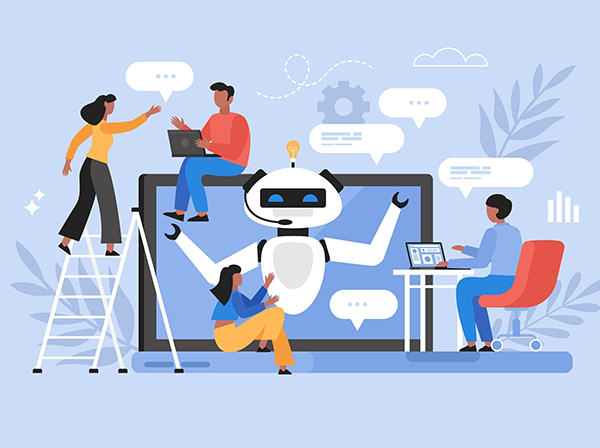
Synthetic intelligence (AI) has witnessed exceptional progress in recent times, with one in all its most notable achievements being the event of huge language fashions (LLMs). These fashions have revolutionized the sphere of pure language processing (NLP), enabling machines to grasp and generate human-like textual content at an unprecedented scale. LLMs are refined AI techniques educated on giant textual content paperwork.
LLMs are educated to find patterns and constructions for comprehending context, semantics, and grammar intricately. By leveraging advanced algorithms and deep studying (DL) methods, these fashions can generate coherent paragraphs and even total essays that seem indistinguishable from these written by people.
The developments in LLMs have considerably impacted varied domains the place human-machine interplay is essential. From enhancing search engines like google’ accuracy to enhancing digital assistants’ capabilities, these highly effective fashions have demonstrated their potential for remodeling how we talk with know-how.
Nonetheless, the rise of LLMs has additionally raised vital moral issues concerning misinformation or malicious use of generated content material. Within the case of LLMs, placing a steadiness between technological development and accountable AI utilization is paramount.
The Position of LLMs in Language Modeling
Language modeling methods kind the spine of LLMs, enabling exceptional developments in textual content era, textual content comprehension, and speech recognition. These fashions are educated to grasp and predict human language patterns by studying from huge quantities of textual information.
Textual content era is a key utility of language fashions. By coaching on various sources similar to books, articles, and on-line content material, these fashions develop the power to generate coherent and contextually related textual content.
LLMs improve the human capacity to extract significant data from written texts. Furthermore, the applying of LLMs extends to speech recognition know-how. By leveraging a eager understanding of spoken phrases and their contextual relationships with different phrases inside sentences or conversations, these fashions contribute to correct transcription techniques that convert spoken phrases into written textual content.
Textual content Classification and Semantic Understanding
Massive language fashions additionally excel in textual content classification duties. They will precisely categorize paperwork primarily based on their content material or sentiment evaluation by successfully capturing nuanced semantic data from the textual content. This distinctive functionality allows companies to automate processes like content material moderation, e-mail filtering, or organizing huge doc repositories.
One other exceptional side is their capacity to understand semantic understanding throughout totally different languages. By coaching on multilingual corpora, these fashions purchase a broader information base that permits them to grasp and generate textual content in a number of languages.
Contextual Data for Improved Efficiency
One key issue that contributes to the spectacular efficiency of huge language fashions is their capacity to leverage contextual data.
They seize semantic nuances by recognizing the dependencies between phrases in a sentence and even throughout a number of sentences or paragraphs. This contextual consciousness permits them to generate extra contextually applicable responses whereas minimizing ambiguity.
LLMs profit from pre-training and fine-tuning methods that refine their understanding of context-specific data. Pre-training entails exposing the mannequin to a variety of duties with huge quantities of unlabeled information, enabling it to amass normal linguistic information.
Wonderful-Tuning Massive Language Fashions for Precision
Wonderful-tuning fashions for precision is a vital course of in adapting LLMs for particular duties, similar to language translation. Whereas pre-trained fashions like GPT-3 possess a exceptional capacity to generate coherent and contextually related textual content, fine-tuning ensures that these fashions can carry out with even larger accuracy and proficiency in focused purposes.
The method of fine-tuning begins by choosing a pre-trained mannequin that finest aligns with the specified job. For instance, if the target is to translate textual content between languages, a mannequin beforehand educated on various multilingual information could be chosen as the start line. Subsequent, the mannequin is additional refined by coaching it on domain-specific or task-specific datasets. Throughout fine-tuning, the mannequin’s parameters are adjusted by iterative optimization methods. By exposing the mannequin to labeled examples from the particular job at hand, it learns to make predictions that align extra intently with floor reality.
This adaptation course of permits the mannequin to generalize its information and enhance its efficiency in areas the place it initially lacked precision. Wonderful-tuning additionally allows customization of fashions primarily based on elements similar to vocabulary or type preferences. By adjusting some particular parameters throughout this course of, practitioners can management how a lot affect pre-existing information has over new duties.
The Impression of NLP, ML, and DL on Massive Language Fashions
NLP, ML, and DL kind the spine of huge language fashions.
NLP is a subfield of laptop science that focuses on enabling machines to grasp and course of human language. It entails varied methods similar to tokenization, part-of-speech, and so forth.
DL is a subfield of ML that employs synthetic neural networks with a number of layers. These networks be taught hierarchical representations of information by progressively extracting higher-level options from uncooked enter.
DL has revolutionized NLP by enabling fashions like GPT-3 to deal with advanced language duties. LLMs prepare on huge quantities of textual content information to be taught the statistical properties of human language. They encode this data into their parameters, permitting them to generate coherent responses or carry out different duties when given textual prompts.
Neural Networks and Transformer Fashions
Neural networks kind the muse of LLMs, enabling them to course of huge quantities of textual content information. Neural networks encompass interconnected nodes models organized into layers, with every unit receiving inputs from the earlier layer and producing an output sign that’s handed on to the following layer. The energy or weight of those connections is adjusted by coaching, a course of the place the mannequin learns to acknowledge patterns throughout the information.
Transformer fashions, a particular kind of neural community structure, have revolutionized language processing duties. They launched consideration mechanisms that permit the mannequin to concentrate on related data whereas processing sequences of phrases. As an alternative of relying solely on fixed-length context home windows like earlier fashions, transformers can seize giant “textual contexts” by attending to all phrases in a sentence concurrently.
The important thing innovation in transformers is self-attention, which allows every phrase illustration to think about dependencies with different phrases within the sequence throughout each encoding and decoding levels. This consideration mechanism permits for higher understanding of contextual relationships between phrases and facilitates extra correct language era and comprehension.
Massive Language Mannequin Purposes: From Textual content Completion to Query Answering
OpenAI’s GPT-3 LLMs have garnered vital consideration because of their exceptional capacity to grasp and generate human-like textual content. These fashions have discovered various purposes, starting from textual content completion duties to extra advanced question-answering techniques.
These fashions excel at predicting probably the most possible continuation for a given immediate, making them invaluable instruments for content material era in varied domains. Customers can depend on these fashions to help them in creating coherent and contextually applicable content material, whether or not that’s writing poetry, coding, or drafting emails.
The GPT-3 LLMs have revolutionized query answering by offering extremely correct responses primarily based on supplied queries. By coaching on huge quantities of information from various sources, these fashions possess an in depth information base that enables them to grasp and reply questions throughout varied subjects. This has immense implications for fields similar to buyer assist, schooling, and knowledge retrieval.
Fashions like GPT-3 are pre-trained on giant datasets from the web, permitting them to be taught grammar, details, and even some reasoning skills. Wonderful-tuning then permits these pre-trained fashions to be tailor-made for particular duties or domains. Algorithmic developments have additionally led to enhancements in mannequin efficiency and effectivity. Strategies like distillation allow smaller variations of huge language fashions with decreased computational necessities whereas preserving most of their capabilities.
The Way forward for Massive Language Fashions: Knowledge Ethics
As language fashions proceed to advance and evolve, it turns into essential to handle the moral concerns that come up with their widespread adoption. Whereas LLMs supply immense potential for varied purposes, there are a number of moral issues that want cautious examination.
One key side is the potential for bias and discrimination inside these fashions. Language fashions are educated on huge quantities of information from the web, which might embody biased data and perpetuate present societal prejudices. This raises issues about unintentionally reinforcing stereotypes or marginalizing sure teams.
One other vital consideration is privateness and information safety. Language fashions typically require entry to huge quantities of private information to enhance their efficiency. Nonetheless, this raises questions on consumer consent, information storage practices, and potential misuse of delicate data.
Moreover, accountability and transparency pose vital challenges in the way forward for language fashions. As they grow to be extra advanced and complex, it turns into obscure how choices are made inside these techniques. Guaranteeing transparency in algorithms’ decision-making processes is essential to keep away from any potential manipulation or bias.
Lastly, there’s a concern concerning mental property rights and possession over generated content material by language fashions. As these fashions grow to be extra able to producing artistic works similar to articles or music compositions autonomously, figuring out authorship and copyright laws turns into more and more advanced.
Picture used beneath license from Shutterstock.com
[ad_2]