
[ad_1]
Organizations everywhere in the world – each revenue and nonprofit – are leveraging knowledge analytics for improved enterprise efficiency. Findings from a McKinsey survey point out that data-driven organizations are 23 instances extra more likely to purchase clients, six instances as more likely to retain clients, and 19 instances extra worthwhile [1]. Analysis by MIT discovered that digitally mature corporations are 26% extra worthwhile than their friends [2]. However many firms, regardless of being data-rich, battle to implement knowledge analytics as a result of conflicting priorities between enterprise wants, accessible capabilities, and assets. Analysis by Gartner discovered that over 85% of knowledge and analytics tasks fail [3] and a joint report from IBM and Carnegie Melon exhibits that 90% of knowledge in a corporation isn’t efficiently used for any strategic objective [4].
With this backdrop, we introduce the “knowledge analytics material (DAF)” idea, as an ecosystem or a construction that allows knowledge analytics to perform successfully primarily based on (a) enterprise wants or targets, (b) accessible capabilities akin to individuals/expertise, processes, tradition, applied sciences, insights, decision-making competencies, and extra, and (c) assets (i.e., elements {that a} enterprise must function the enterprise).
Our main aim of introducing the info analytics material is to reply this basic query: “What’s required to successfully construct a decision-enabling system from Information Science algorithms to measure and enhance enterprise efficiency?” The info analytics material and its 5 key manifestations are proven and mentioned under.
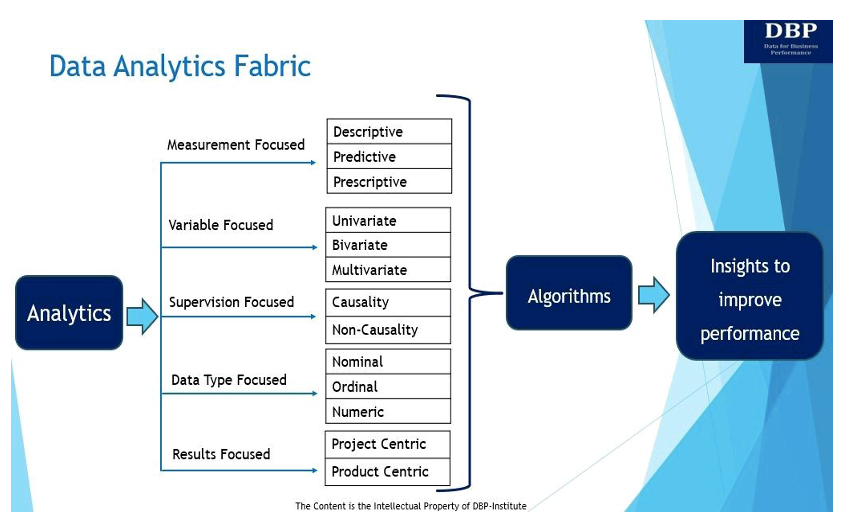
1. Measurement-Targeted
At its core, analytics is about utilizing knowledge to derive insights measure and enhance enterprise efficiency [5]. There are three most important forms of analytics to measure and enhance enterprise efficiency:
- Descriptive analytics asks the query, “What occurred?” Descriptive analytics is used to investigate historic knowledge to establish patterns, tendencies, and relationships utilizing exploratory, associative, and inferential knowledge evaluation strategies. Exploratory knowledge evaluation strategies analyze and summarize knowledge units. Associative descriptive evaluation explains the connection between variables. Inferential descriptive knowledge evaluation is used to deduce or conclude tendencies a few bigger inhabitants primarily based on the pattern knowledge set.
- Predictive analytics seems at answering the query, “What’s going to occur?” Mainly, predictive analytics is the method of utilizing knowledge to forecast future tendencies and occasions. Predictive evaluation may be carried out manually (generally often known as analyst-driven predictive analytics) or utilizing machine studying algorithms (also called data-driven predictive analytics). Both method, the historic knowledge is used to make future predictions.
- Prescriptive analytics helps to reply the query, “How can we make it occur?” Mainly, prescriptive analytics recommends one of the best plan of action for shifting ahead utilizing optimization and simulation strategies. Sometimes, predictive evaluation and prescriptive analytics go collectively as a result of predictive analytics helps discover potential outcomes, whereas prescriptive analytics seems at these outcomes and finds extra choices.
2. Variable-Targeted
Information may also be analyzed primarily based on the variety of variables accessible. On this regard, primarily based on the variety of variables, the info analytics strategies may be univariate, bivariate, or multivariate.
- Univariate Evaluation: Univariate evaluation includes analyzing the sample current in a single variable utilizing measures of centrality (imply, median, mode, and so forth) and variation (normal deviation, normal error, variance, and so forth).
- Bivariate Evaluation: There are two variables whereby the evaluation is said to trigger and the connection between the 2 variables. These two variables may very well be dependent or unbiased of one another. The correlation method is essentially the most used bivariate evaluation method.
- Multivariate Evaluation: This system is used for analyzing greater than two variables. In a multivariate setting, we sometimes function within the predictive analytics area and a lot of the well-known machine studying (ML) algorithms akin to linear regression, logistic regression, regression bushes, assist vector machines, and neural networks are sometimes utilized to a multivariate setting.
3. Supervision-Targeted
The third sort of knowledge analytics material offers with coaching the enter knowledge or unbiased variable knowledge that has been labeled for a selected output (i.e., the dependent variable). Mainly, the unbiased variable is the one the experimenter controls. The dependent variable is the variable that adjustments in response to the unbiased variable. The supervision-focused DAF may very well be certainly one of two sorts.
- Causality: Labeled knowledge, whether or not generated mechanically or manually, is important for supervised studying. Labeled knowledge permits one to obviously outline a dependent variable, after which it’s a matter of the predictive analytics algorithm to construct an AI/ML device that will construct a relationship between the label (dependent variable) and the set of unbiased variables. The truth that we have now a definite demarcation between the notion of a dependent variable and a set of unbiased variables, we permit ourselves to introduce the time period “causality” to greatest clarify the connection.
- Non-Causality: After we point out “supervision-focused” as our dimension, we additionally imply the “absence of supervision,” and that brings the non-causal fashions to the dialogue. The non-causal fashions deserve point out as a result of they don’t require labeled knowledge. The fundamental method right here is clustering, and the preferred strategies are k-Means and Hierarchical Clustering.
4. Information Kind-Targeted
This dimension or manifestation of the info analytics material focuses on the three several types of knowledge variables associated to each the unbiased and dependent variables which are used within the knowledge analytics strategies for deriving insights.
- Nominal knowledge is used for labeling or categorizing knowledge. It doesn’t contain a numerical worth and therefore no statistical calculations are potential with nominal knowledge. Examples of nominal knowledge are gender, product description, buyer tackle, and the like.
- Ordinal or ranked knowledge is the order of the values, however the variations between each shouldn’t be actually identified. Frequent examples listed here are rating firms primarily based on market capitalization, vendor cost phrases, buyer satisfaction scores, supply precedence, and so forth.
- Numeric knowledge wants no introduction and is numerical in worth. These variables are essentially the most basic knowledge sorts that can be utilized to mannequin all forms of algorithms.
5. Outcomes-Targeted
Such a knowledge analytics material seems on the methods through which enterprise worth may be delivered from the insights derived from analytics. There are two methods through which enterprise worth may be pushed by analytics, and they’re by way of merchandise or tasks. Whereas merchandise might have to deal with extra ramifications round person expertise and software program engineering, the modeling train finished for deriving the mannequin can be related in each the challenge and product.
- A knowledge analytics product is a reusable knowledge asset to serve the long-term wants of the enterprise. It collects knowledge from related knowledge sources, ensures knowledge high quality, processes it, and makes it accessible to anybody who wants it. Merchandise are sometimes designed for personas and have a number of lifecycle phases or iterations at which product worth is realized.
- A knowledge analytics challenge is designed to deal with a selected or distinctive enterprise want and has an outlined or slender person base or objective. Mainly, a challenge is a short lived endeavor supposed to ship the answer for an outlined scope, inside finances and on time.
The world’s economic system will dramatically rework within the coming years as organizations will more and more use knowledge and analytics to derive insights and make choices to measure and enhance enterprise efficiency. McKinsey discovered that firms which are insight-driven report EBITDA (earnings earlier than curiosity, taxes, depreciation, and amortization) will increase of as much as 25% [5]. Nevertheless, many organizations should not profitable in leveraging knowledge and analytics for enhancing enterprise outcomes. However there isn’t any one normal method or method to ship knowledge analytics. The deployment or implementation of knowledge analytics options is determined by enterprise targets, capabilities, and assets. The DAF and its 5 manifestations mentioned right here can allow analytics to be deployed successfully primarily based on enterprise wants, accessible capabilities, and assets.
References
- mckinsey.com/capabilities/growth-marketing-and-sales/our-insights/five-facts-how-customer-analytics-boosts-corporate-performance
- ide.mit.edu/insights/digitally-mature-firms-are-26-more-profitable-than-their-peers/
- gartner.com/en/newsroom/press-releases/2018-02-13-gartner-says-nearly-half-of-cios-are-planning-to-deploy-artificial-intelligence
- forbes.com/websites/forbestechcouncil/2023/04/04/three-key-misconceptions-of-data-quality/?sh=58570fc66f98
- Southekal, Prashanth, “Analytics Greatest Practices”, Technics, 2020
- mckinsey.com/capabilities/growth-marketing-and-sales/our-insights/insights-to-impact-creating-and-sustaining-data-driven-commercial-growth
[ad_2]